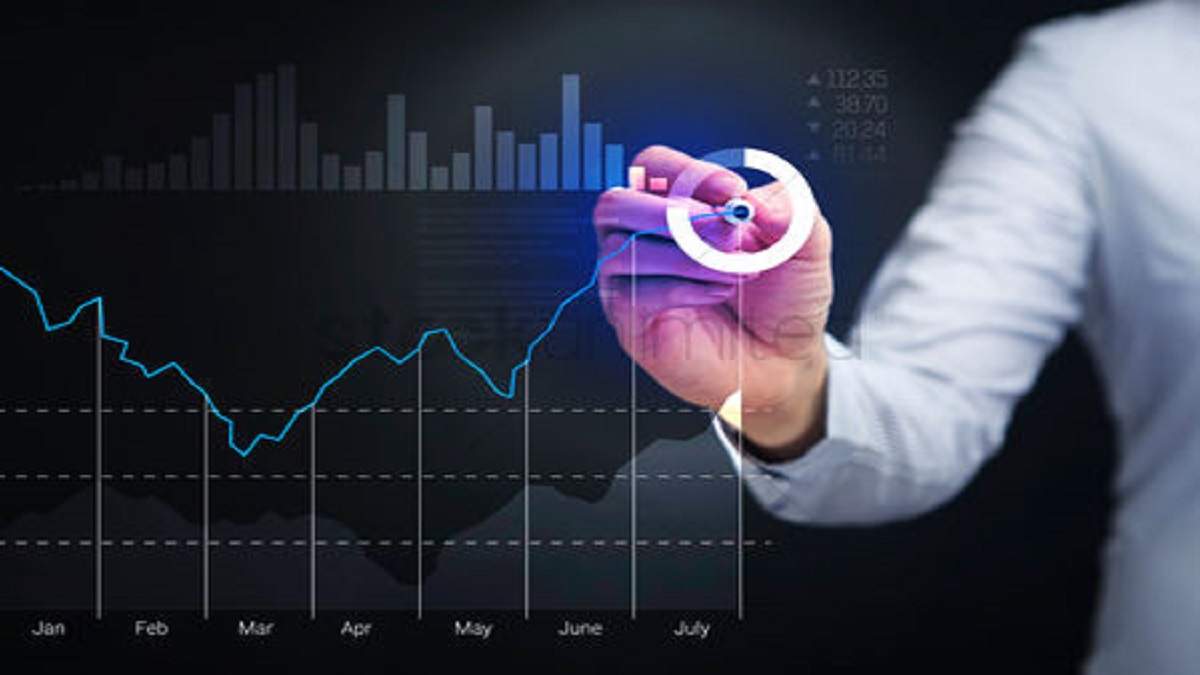
Accurate. Relevant. Consistent. Data Quality Assessment (DQA) Is More Critical Than Ever
Data is the lifeblood of today’s enterprises.
Sales, marketing, finance, HR — every branch of an enterprise relies on data in some way to make critical decisions.
The problem is, the magnitude of data we generate today is of extraordinary proportions, 2.5 quintillion bytes every day, according to IBM’s estimate. That is approximately 2.5 billion gigabytes. In terms of size, if one gigabyte is the volume of Earth, 2.5 billion gigabytes represent nearly 770 suns.
That’s a lot.
Given that, how do we figure out what’s junk and what’s not?
To enterprises, data is more critical than ever. And so is data quality assessment.
What is Data Quality Assessment (DQA)?
In the US alone, poor quality data costs us over $3 trillion (Accenture).
How?
Riddled with errors, redundancies, and irrelevant information, poor quality data misleads us into making inaccurate business decisions.
The solution is to make regular data quality assessments — following a defined set of guidelines to sort and cleanse enterprise data, ensuring that it is free of errors, redundancies, and irrelevant information.
What’s left is high-quality data that enables enterprises to make more accurate, more effective decisions.
In fact, adopting DQA has multiple, far-reaching benefits. It ensures that data is —
- Accurate, perfecting targeted advertising, for example
- Relevant, saving enterprises ample time and money
- Complete and consistent, ensuring also the completeness and consistency of data-based decisions
- Timely, allowing enterprises to identify the right opportunities at the right time
So, how does one get started with DQA?
How is a Data Quality Assessment (DQA) made?
In simple terms, a DQA is made by comparing the data you have with the data you ought to have.
The data you have is collected from a wide range of sources, as mentioned, like sales, marketing, finance, and HR. The data you ought to have is determined by an established set of rigid DQA guidelines.
Of course, it’s not that simple.
Enterprises grapple with several complex questions before a DQA process is established. Who decides the guidelines? And how? What does high-quality even mean?
Sticking to simplicity for now, enterprises can get started with DQA by following the 3 steps.
1. Lay the foundation
The first step is to determine the scope of the data your enterprise can access. Then, develop a framework to collect, sort, and manage the data efficiently.
Every enterprise has unique needs, and so should be the framework one develops.
To identify their unique requirements, enterprises ask themselves questions like,
- Which team or individual will have access to the data?
- What is their goal?
- How flexible is their workflow?
Remember that efficiency and efficacy are equally vital. Develop. Then, optimize.
2. Clean
Once the scope is identified, one can start collecting data.
Once the data is collected, it must be thoroughly monitored and analyzed. The goal is to validate it against the established framework.
As the data passes through the rule-based guidelines, errors emerge, like duplication, incorrect formatting, redundancies, and so forth.
What’s left at the end is data that is accurate, complete, consistent, and valid.
3. Keep it going
Data Quality Assessments (DQA) are not one-and-done processes. They must occur regularly to ensure that nothing slips through the gaps.
The importance of data governance solutions
Two challenges.
The first step to implement DQA is also the most difficult step to implement DQA. But it is also the most crucial.
Laying a foundation is awfully time-consuming. It demands meticulous planning, asking managers and leadership to really and deeply understand their process workflow.
But it’s for their own good. Since to get the most out of DQA, frameworks must fit process workflows like a glove. Once the foundation is in place, the rest is relatively easy.
The second hardest challenge is to keep it going. It’s to prepare for and manage change. To adapt or readjust your framework to it, time and again.
Now, enterprises can invest enormous time and energy to understand their own ins and outs and build a robust framework on that basis.
On the other hand, they can get in touch with world-class providers of data analytics solutions like SG Analytics, who offer data governance solutions tailor-made for their unique needs, enabling comprehensive and consistent data quality assessment in the future.
If you’re going to do it, do it right.